Refraction AI REV-1
How user-centered research led to 2X-ing customer acquisition in 6 months
Just so that you know what exactly the REV-1 is…here's a video of it in action.
As a customer places an order at a partnered restaurant, the order gets routed to Refraction AI, which dispatches the autonomous self-driving robot to the restaurant, which then delivers it to the customer for a flat delivery fee.
Details and deliverables for the REV-1 are protected under NDA, and has since been discontinued as development shifted towards the second generation of the product (REF-1), thus I will only be using this as an opportunity to talk about my contributions without any artifacts.
During COVID, Refraction AI was gaining traction in the autonomous delivery space, raising about $10 million as a startup based in Ann Arbor, my hometown.
As an early-stage startup, my role on the product development team had overlapping responsibilities in various methods of research and data collection. Here's the inside scoop on how this hands-on approach led to a staggering doubling of customer acquisition in just six months for the Rev-1.
Role
Product Development Associate, R&D + Operations
Collaborators
Various engineers and other PDAs.
Timeline
~6 months (2021 Q1-Q3)
Problem
As an early-stage startup, Refraction AI was developing their first fleet by prioritizing technical feasibility, but lacked in some more nuanced areas, requiring additional research.
We faced various challenges with the REV-1, impacting customer perception and delivery performance.
Major Issues
Public trust
Pedestrians, drivers, customers, and restaurant clients all shared skepticism around self-driving robots.
Reliability
Delivery times and and costs were generally comparable to substitute delivery methods (ex: DoorDash or Uber Eats), but were not as consistent or reliable.
Interaction dynamics
The REV-1 robot ran into many unforeseen scenarios and circumstances with people, traffic, weather, and the countless factors in the real-world.
How might we…
With a vast amount of quantitative data our engineers could collect from our internal platforms, there's still a mountain of unexplored qualitative data that needed to be collected, which could hopefully give us insights that answered our problems.
How might we generate actionable insights that can lead to more scalable, sustainable, and profitable REV-1 operations.
Solution
Over the course of 6 months, we collected an extensive set of qualitative data on our users, the robot's performance, and other observations, which I used to collaborate with engineers to implement improvements in safety protocols, smarter routing, procedural/operational efficiencies, and new features.
I translated hundreds-of-hours of qualitative data into insights, which powered the implementation of multiple procedural efficiencies, and improvements in various problem areas, doubling customer acquisition in 6 months.
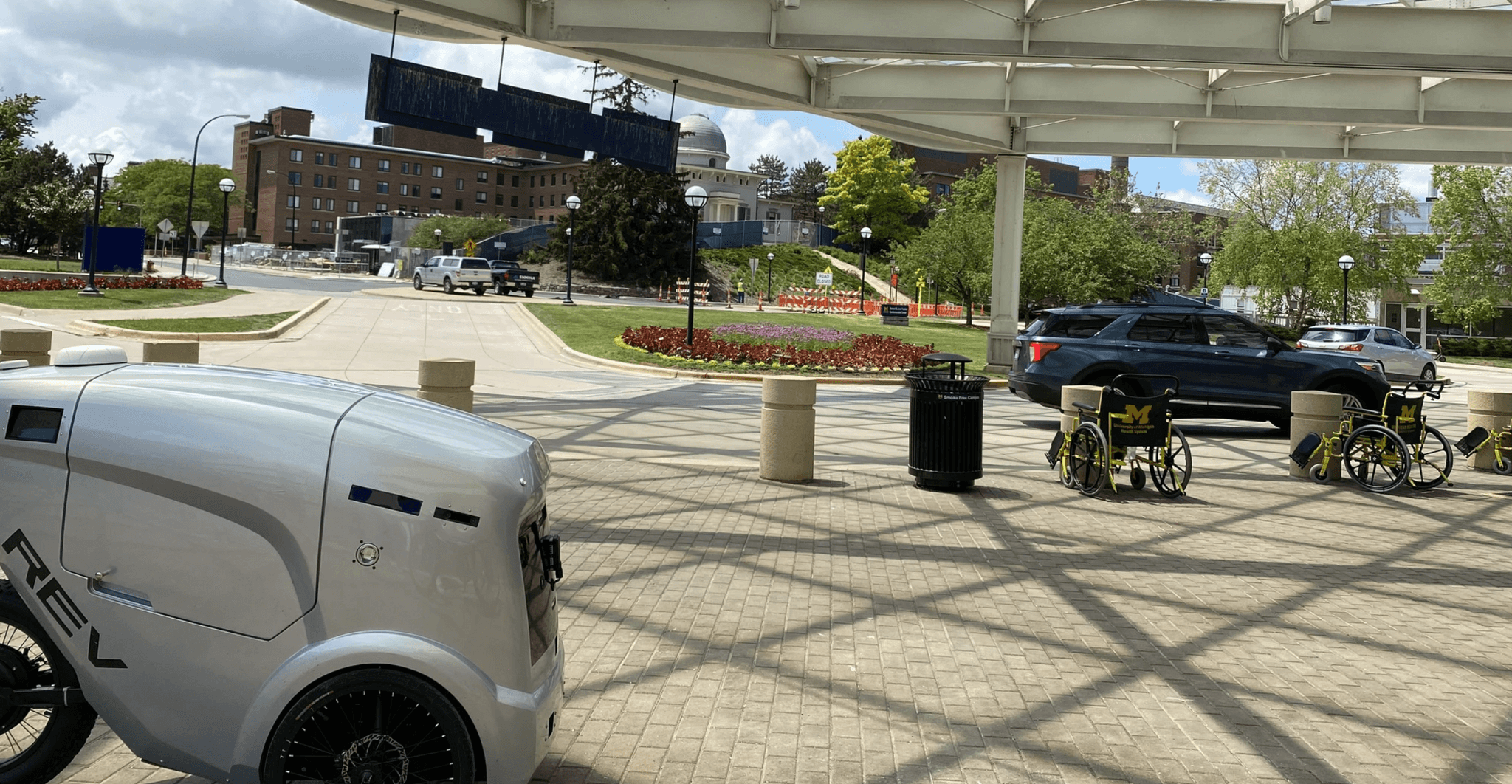
Impact
Measuring success
We measured success by observing these changes over time after the new implementations.
KPIs
~30%
Outbound/Delivery Times
A large portion of delivery times were inflated by delays from the fleet hub, prior to even arriving at a restaurant. Optimizing procedures to decrease outbound delays led to a decrease in overall delivery times.
~20%
Average Routing Efficiency
Routing efficiency was dramatically improved for peak-demand intervals like high-pedestrian-level times/days.
2x
Customer Acquisition
Although not all new customers can be attributed to operation improvements, other indirect factors like customer awareness and perception as a result of new features helped double the amount of restaurants we were delivering for, and by proxy, the amount of customers receiving food deliveries via REV-1.
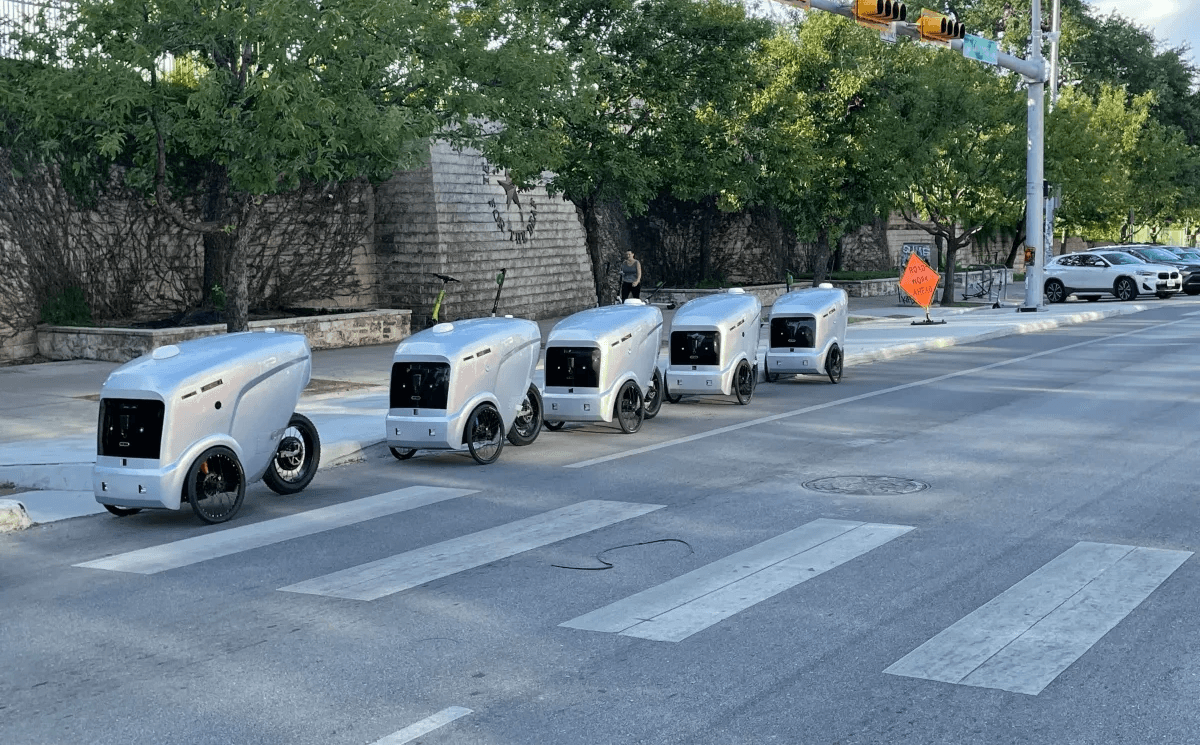
Field Research
I shadowed the REV-1 on an electric scooter with a GoPro attached to gather unfiltered, real-time data. I closely observed how the robot navigated its environment—whether it was maneuvering around a pothole or pausing for a pedestrian. These raw moments, captured on my GoPro, revealed insights far deeper than any data graph could. This ‘boots-on-the-ground’ approach immersed me directly in the product’s real-world application.
By following deliveries in real-time over several months, I was exposed to various scenarios and interactions with people and the environment.
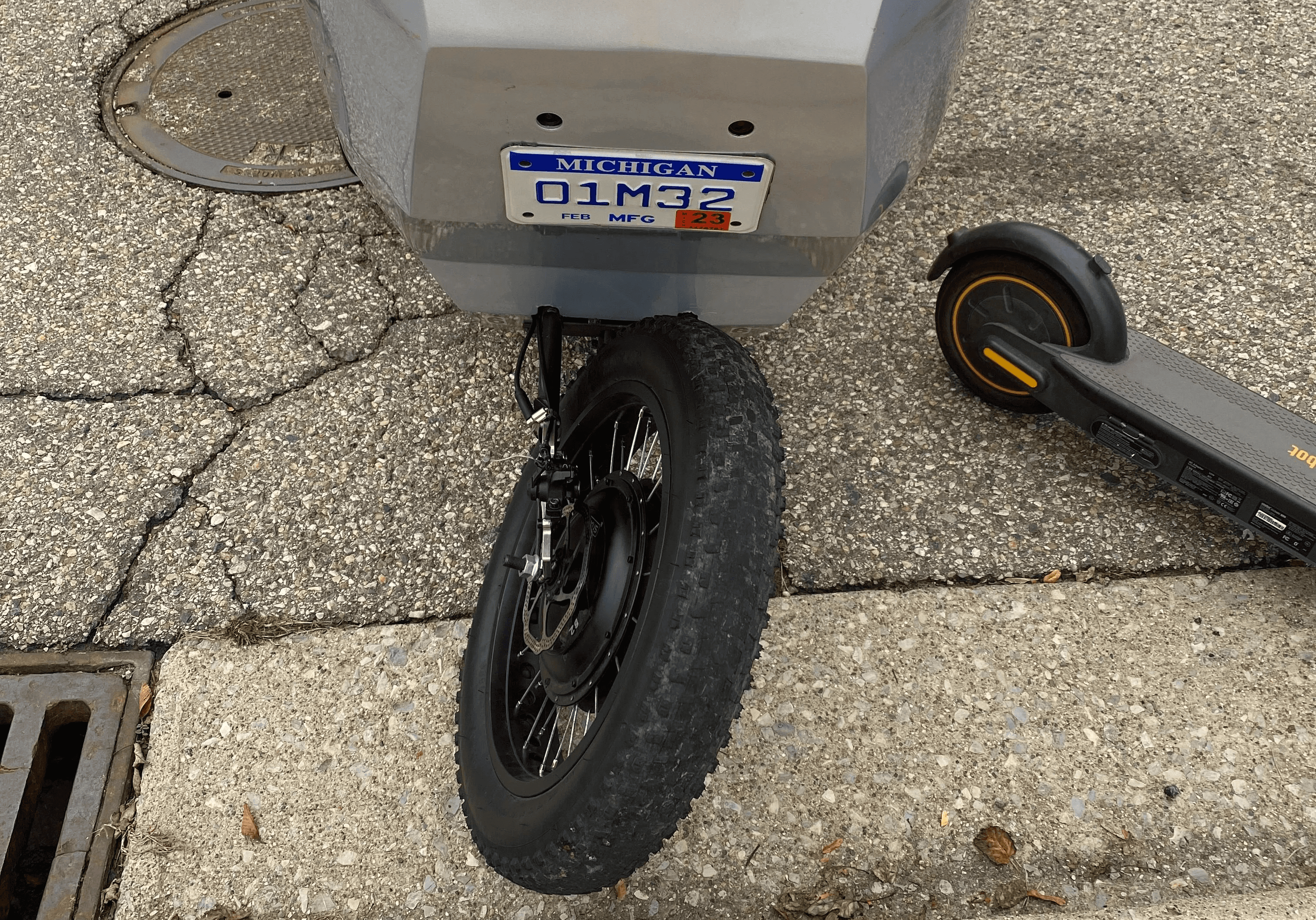
Here's when we ran into a pothole and the robot couldn't complete a delivery.
In-situ interviews
Beyond the high-speed pursuits, I made it a priority to connect with restaurants and customers. Given the hustle of city life, most people were on the move—and the robot had deliveries to make, too. These interactions were brief, and so my objective was clear: to extract valuable insights from these fleeting moments. How did they feel about seeing the robot? Were they satisfied with delivery times? Did they trust this autonomous delivery service?
As I wrapped up these quick exchanges, it became evident that reactions to the Rev-1 robot were mixed. Some users were genuinely enthusiastic, impressed by the innovation, even snapping photos to share with friends. But many were still skeptical. Some questioned the reliability and safety of autonomous delivery, while others were more blunt in their reservations—one driver even flipped me off in disapproval (ironically, while driving a Tesla, which I found funny). These diverse responses underscored the range of public perceptions, highlighting the need not only for technical refinement but possibly also for community engagement and awareness.
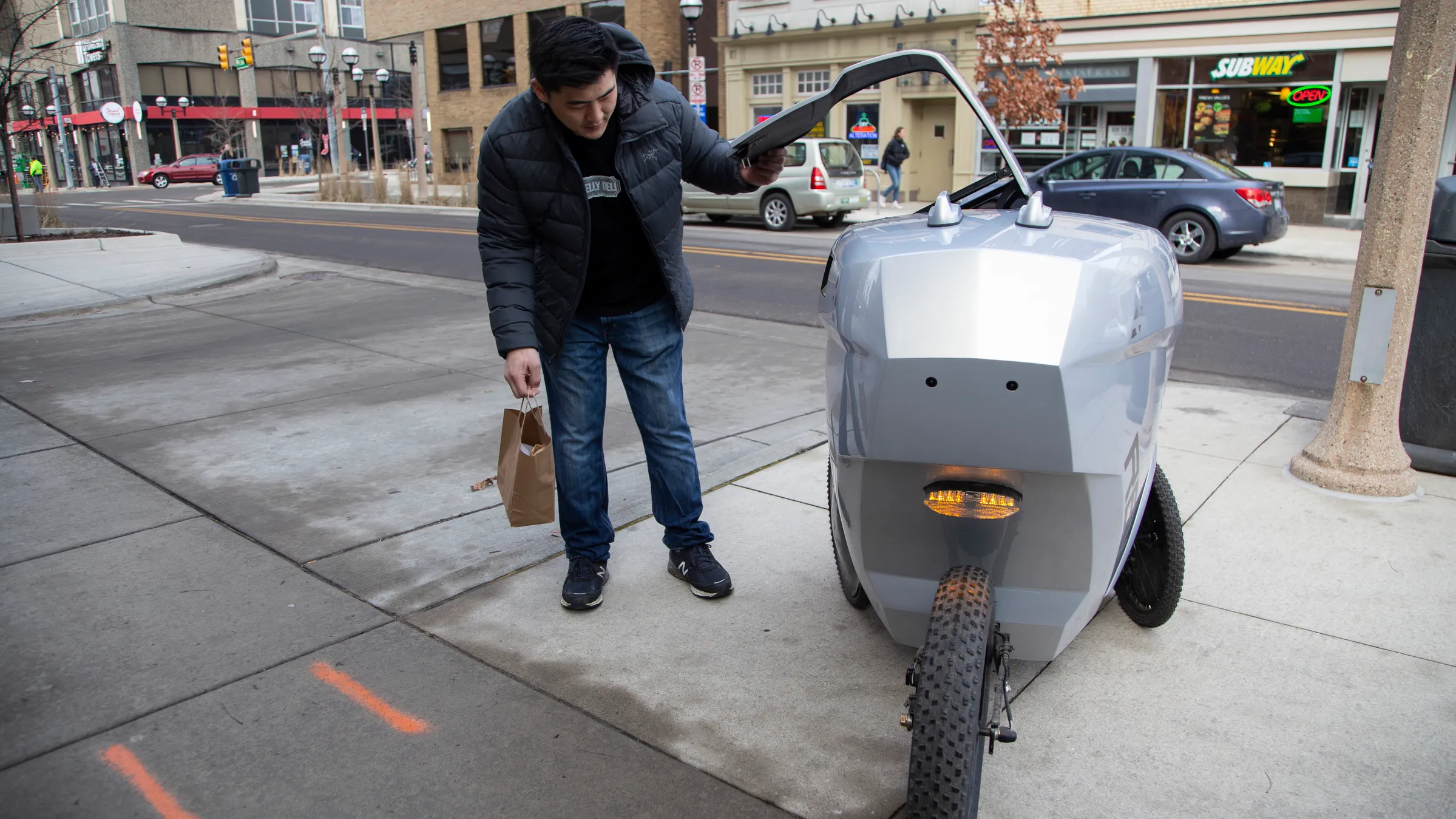
Key Insight 1: Trust is a big deal
Despite the robot's advanced tech, some folks were understandably concerned about safety. And while I was equipped with an electric E-stop button on my scooter as a safety net, it was evident we needed to do more to build public trust.
Key Insight 2: The familiarity factor
While the public's trust was a recurring theme, another facet of trust needed addressing — the restaurant workers' familiarity with the system. Many were still getting to grips with how the robot functioned, leading to occasional hiccups during the food handover.
Key Insight 3: No one likes to wait
It wasn’t just about robot deliveries; it was about fast robot deliveries. Every minute counted, and we needed to ensure the robot's pathfinding was on point. the process from order receipt to delivery completion required more fluidity. Whether it was the robot's hesitation in unfamiliar terrains or the staff's uncertainty about interactions, a streamlined approach was crucial.
Key Insight 4: Curiosity can't be prevented
The robot's novelty naturally drew attention. People would often halt me/the robot, hungry for information or a quick chat about the robot. While their interest was heartening, these unexpected pauses invariably impacted delivery times, especially during busy pedestrian-heavy days like game days at the University of Michigan.
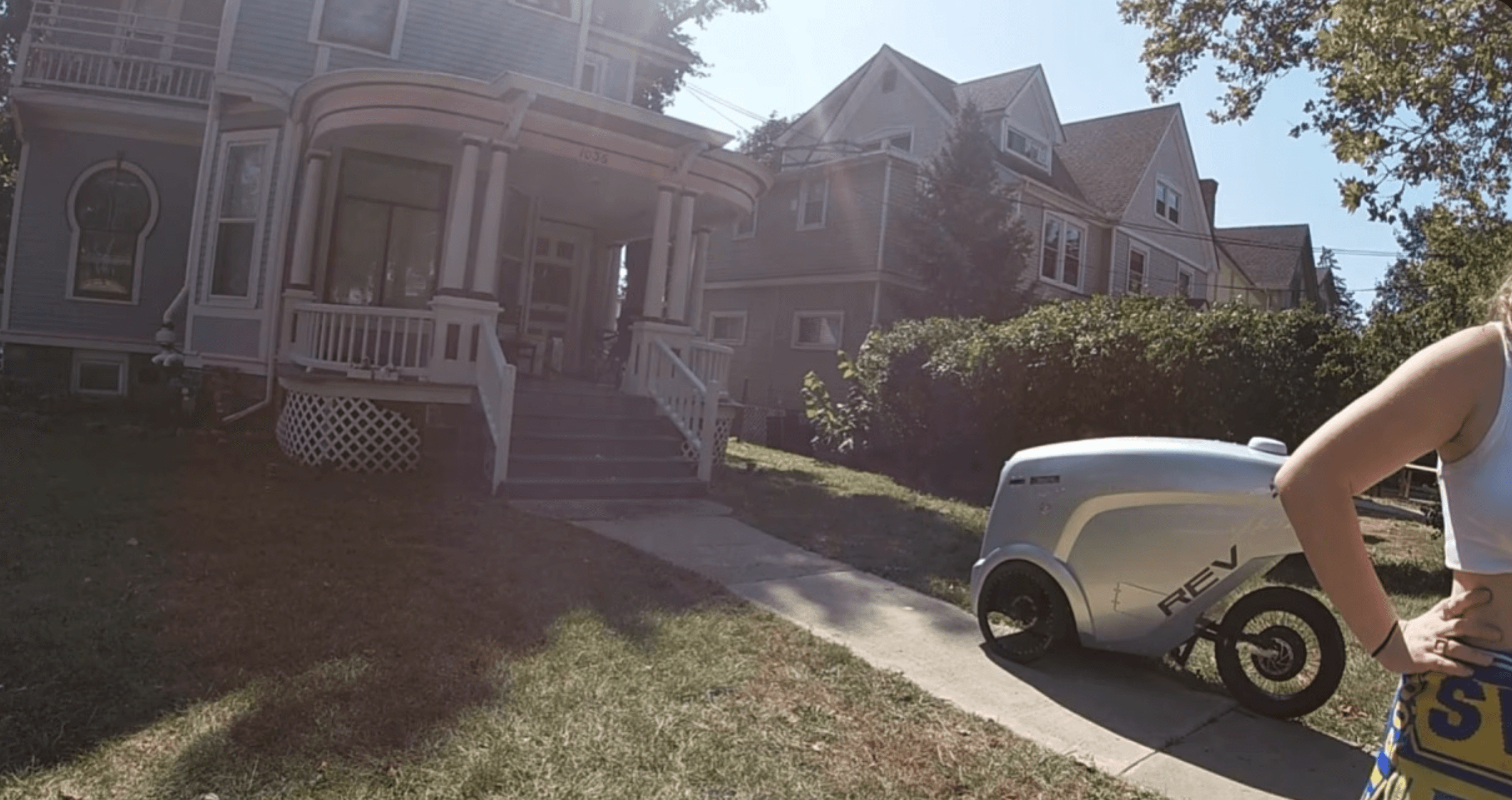
Product Implementations
As my contributions were integrated into Refraction AI's operations, we started to observe notable shifts. the immediate effects weren’t necessarily in surges of new customers, but rather in the consistency with which existing and new customers engaged with our services.
One unmistakable byproduct was the increase in completed deliveries. With streamlined safety checks and improved outbound times, the Rev-1 robot was spending less time in prep and more time on the road. This efficiency meant we could cater to more orders within the same timeframe. As a result, we not only satisfied our existing customer base but also showcased our capability to potential new customers, gradually boosting our customer acquisition.
My insights led to multiple new optimizations and implementations, both big and small, which translated into tangible results, proving that attention to user experience directly impacted business growth.
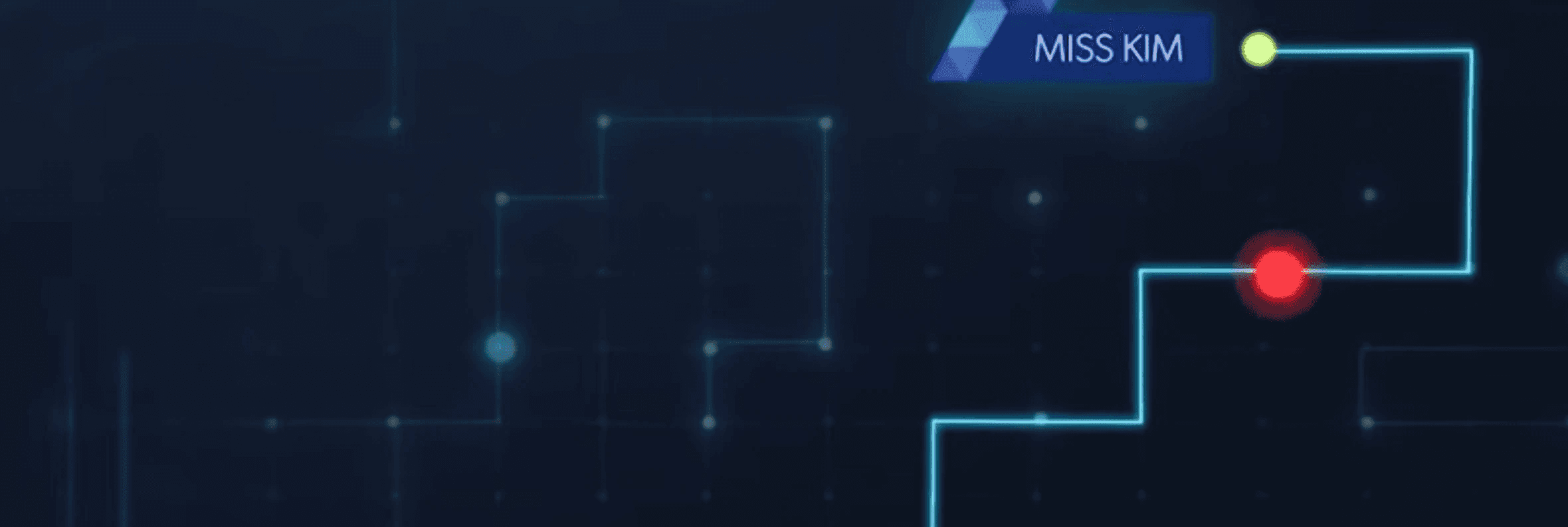
Solution 1: Safety first (and fast)
At Refraction AI, I prioritized safety without sacrificing efficiency. I observed that while safety checks were crucial, there was room for streamlining. I developed a quick-reference guide for our internal digital checklists, and introduced a protocol to have equipment prepped before delivery calls. These initiatives minimized response times and ensured the robot was always ready for a seamless, safe delivery experience.
Solution 2: Smarter routes, not just faster ones
The data showed that the shortest route wasn’t always practical for the Rev-1 robot. While using the Google Maps API for route optimization, we found that pedestrian-heavy areas and signal interference in certain zones caused frequent stops and delays. My on-the-ground observations helped us identify these issues, leading to refinements in the robot's routing algorithms for smoother navigation through real-world challenges.
Solution 3: Harnessing curiosity, not avoiding it
We quickly identified the inherent curiosity the robot ignited in passersby. After I moved on and left Refraction AI, the team took this observation to the next level. They enhanced the robot with interactive features, turning the delivery process into a more engaging experience. Additionally, they introduced advertising campaigns onto the robot's exterior. This move cleverly capitalized on the attention the robot naturally attracted, turning the Rev-1 into a mobile billboard, simultaneously promoting brands while executing its delivery duties.
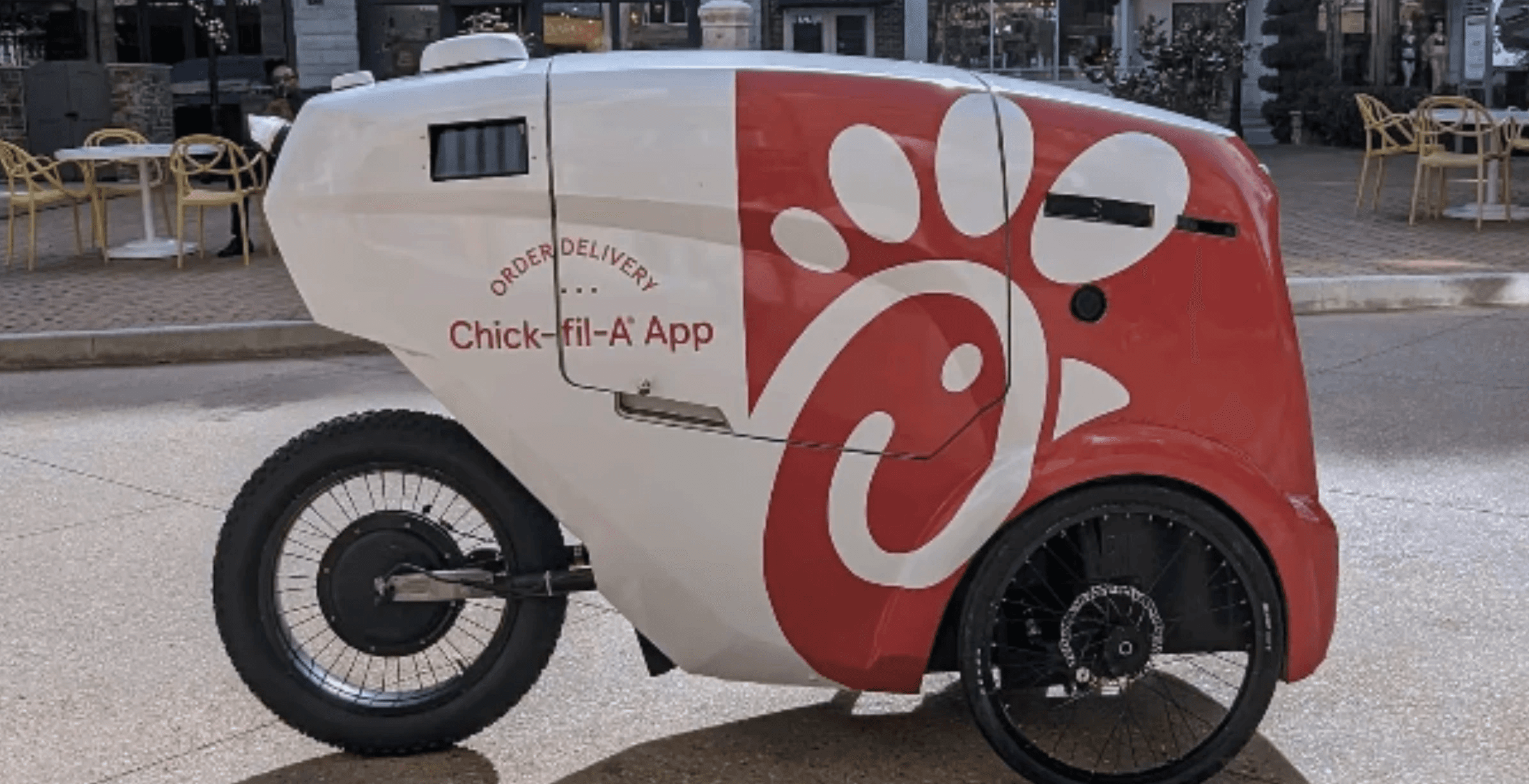
What I Learned
Reflecting on my time at Refraction AI, I realize how invaluable that hands-on experience was. It wasn’t just about collecting data; it was about understanding people and their behavior. As I've since transition into design, these lessons on empathy, observation, and action still remain etched in my mind as I work on various products. In a world driven by technology, it's the human touch that makes all the difference. and my scooter-riding days with the REV-1 robot are a testament to that.
✦
✦
Contact Me
Currently based in Atlanta, GA
but I love moving around.
You made it this far, so we might as well talk.
I promise it's worth it.
Designed and built by Kevin Esmael Liu, 2024 | No rights reserved—steal whatever you want bc I'll just come up with something better.